In Episode 74 of The Redirect Podcast, we’re joined by Britney Muller, formerly at HuggingFace and Moz, to discuss the implications of generative AI technologies and large language models used by Open.AI’s ChatGPT and Google’s Bard. We deep dive on not only the pros and cons of using AI for marketing, but explore proposed guardrails and guideposts that might need to be in place. Stick around for the bonus on the future of AI toward the end!
Breaking Down Generative AI
Simply stated, Generative AI is artificial intelligence that has the ability to create content without much human intervention. Content created by generative AI could be inclusive of written text, images, videos, and even music. The most discussed as of recent are OpenAI’s ChatGPT and Google’s Bard.
Common Uses of Generative AI Include:
- Text-based content generation that’s written in a natural and more conversational approach.
- Realistic image creation and renderings of product design.
- Music creative based off of original music samplings, remixes, etc.
- Code & Programming needs for strings of code from regex to PHP.
What are Large Language Models Based Off Of?
Large Language Models (LLM) are a form of an artificial intelligence (AI) algorithms used to process and understand large sets of data and deliver a prediction of new content to be created. With machine learning, there is general intelligence and narrow intelligence – with most of AI residing in the latter. In meaning, we as humans train a model to do something that’s very specific. Examples that Britney provided are identifying precancerous cells and different medical paths. General intelligence is a lot wider, where you can ask a model to do nearly anything.
How Long has Generative AI Been Around?
Generative AI isn’t new — many of these models have been around since the 1960’s. As Britney points out in her research, most of AI is rooted in statistics. Take, for example, handwritten digits, which can be related closely to the “Hello World” (programming reference), of the machine learning landscape and is referred to as MNIST. MNIST, or Modified National Institute of Standards and Technology, is the large database of handwritten digits commonly used for training image systems used in the field of machine learning.
Consider the digits 1-2-3. These are basic digits that have been written out a number of times and we might want to identify and classify those numbers as they are written. This is done by using models that train, over time, and cluster these elements together. All of this happens in a multidimensional space that humans have an extremely difficult time visualizing. The clustering of these digits in their respective space, is what helps map out and create a latent understanding of language and how things are connected to one another.
What makes ChatGPT and Large Language Models Special?
All kidding aside, not much makes AI special but statistics, just on a large scale. Truth be told, as Britney explains it in the podcast – “LLMs, especially ChatGPT, are simply predicting one word at a time. What’s unique about it, is that it has trained on all this world’s information and it knows the next probable word and to what degree it needs to be used.”
An Example from ChatGPT
Prompting ChatGPT to provide the probability of the next word to be used in a sentence. The example being: “A camper reported which bear the girl had [ blank ]”
Note: Britney received completely different responses and scores
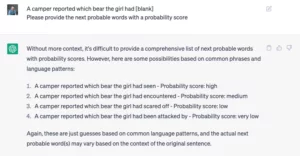
From a probability perspective, this is where things get interesting. If you had the model generate the highest, most probable next word every time, it would be boring and repetitive. On the contrary, you don’t also want it to draw words out of a hat – meaning, you have to find what that random sampling is that creates a sweet spot. Within some models, these are more like temperature settings. The hotter the temperature, the language might get more creative and expansive.
Should Guideposts be Implemented on Generative AI?
Furthering the conversation beyond what the AI models are based off of and their uses, the bigger question comes up around the general public’s (and marketer’s) use of AI. Tools such as ChatGPT could be taken out of context and used verbatim with no guardrails or checks and balances in place.
Our stance is — as Britney also concurs — that you do have to fact check what is coming out of the tool; consider it your smartest friend in the room who isn’t perfect. Ethically speaking, especially for those of us in the marketing and communications field, we really do need to consider the output of generative AI tools and their place in our process flow.
Who is Leading AI Ethics & Research?
No matter your stance on using generative AI for your marcom purposes, if you’re interested in exploring more of the conversations that are being had on the ethical use and output of AI, consider giving the following individuals a look before you take much of what’s being put out there by mainstream publishers. These are highly intelligent and well respected individuals in their field:
Margaret Mitchell – AI Research Scientist and Founder of Ethical AI. Typically their research is on the subjects of vision-language and grounded language generation.
Emily Bender – Professor in the Department of Linguistics at The University of Washington and Director of the Computational Linguistics Laboratory.
Timnit Gebru (DAIR) – Founder & Executive Director of the Distributed Artificial Intelligence Research Institute (DAIR), and former Googler co-lead the Ethical AI research team.
We’ll continue to update this post as elements in the AI space are advancing at light speed. Please give our special guest, Britney Muller, a follow on Twitter, and take a look at some of our recent articles and podcasts on AI and SEO.
Leveraging ChatGPT for SEO: Content Marketing Implications
BTW with BTM Podcast: ChatGPT, Google Bard & The Impact AI will have on SEO